With good cause, the benefits of large language models, or LLMs, are causing a stir in the field of artificial intelligence. However, what precisely gives these models their strength?
The truth is that LLMs can comprehend and produce content that is human-like, surpassing the capabilities of conventional linguistic solutions. They also provide hitherto unheard-of chances to enhance consumer satisfaction and optimize operations. Let’s examine the advantages of big language models and how they might support your objectives.
Some Advantages of LLM For Business
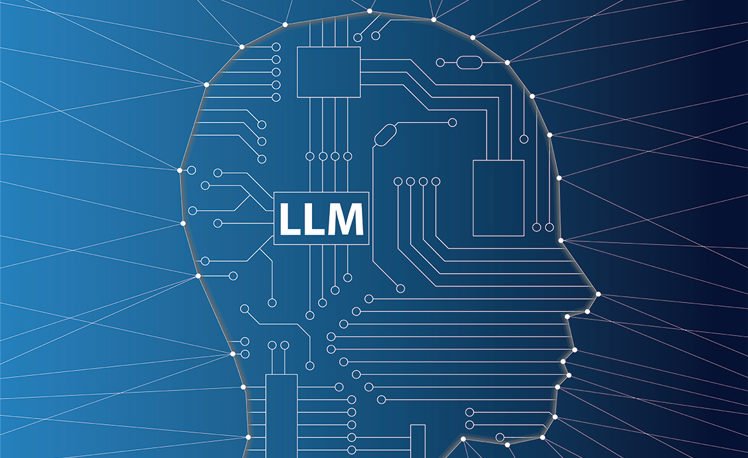
1. Simplifying Procedures
Streamlining processes, which includes automating monotonous procedures, increases productivity, lowers overhead expenses, and improves efficiency. Salesforce is the ideal illustration of this. The business processes enormous volumes of data using the benefits of large language models for analytics and customer relationship management.
They can now devote more time to growing their company by reducing the time they would typically spend on analytics and client interaction. Additionally, this guarantees quicker reaction times.
Salesforce simultaneously changes its operations on a wide scale, increases customer happiness, and improves delivery without compromising efficiency and productivity. In certain particular jobs, human error and intervention are also minimized or eliminated.
2. Product Development Innovation
Product development innovation gives businesses a competitive edge and helps them remain ahead of the competition. LLMs, for instance, can evaluate enormous data sets, spot new patterns, provide insightful information about customer preferences, and reveal ways to enhance current goods and services.
This might assist businesses in creating new items or improving their current offerings. One of the most well-known businesses that has improved its product development by integrating machine learning and sophisticated data analysis is IBM. By forecasting the market and customizing its goods based on trends, IBM obtains a competitive edge.
This helps businesses reduce their marketing expenses and time in addition to increasing their return on investment and making their products/offers more relevant. In conclusion, this advantage goes beyond just enabling businesses to develop more quickly. Innovation is also much more successful when it makes use of insights regarding the requirements of consumers and future expectations.
3. Enhanced Security
By protecting operations and sensitive data from cyberattacks, the answer to how LLMs benefit businesses may contribute to increased security. They may assist in examining network activity, identifying irregularities, and even forecasting security lapses or providing information about regions in need of security enhancements. Mastercard is an excellent illustration of how they elevate real-time protection.
Mastercard employs LLMs to spot fraudulent activity in real time and put preventative measures in place to lower the chance of security breaches and financial crime. This safeguards the business and increases consumers’ faith in its goods and services.
4. Better Production of Content
LLMs are capable of simulating human intelligence and comprehending and learning context from data, both of which may be effectively used to create superior, customized content. This has the potential to greatly increase corporate visibility, tactics, and communication.
Several businesses, like BuzzFeed, employ LLMs to customize content according to customer preferences. This increases BuzzFeed’s success rate and helps it produce material that will engage its audience. Each member of BuzzFeed’s audience receives individualized content, thanks to LLM, which boosts engagement and conversation.
BuzzFeed also aids in streamlining the process of creating content. This enables them to drastically alter the way their content production process operates. In this instance, LLMs contribute to the transformation of content development tactics in dynamic marketplaces.
5. Improved Ability to Make Decisions
The capacity and the benefits of large language models to examine enormous volumes of data may reveal information that human analysts would miss. Businesses may make more strategic and well-informed judgments and get insights that lead to better decisions by putting LLMs into practice.
An outstanding example of this is American Express, which uses LLMs to spot fraud and spending trends. By sorting through transaction data, their LLM helps the business identify fraud in real-time, make better choices sooner, and lower risk.
As a consequence, American Express better manages risk and customizes its offerings to suit consumer preferences. Increased client satisfaction and operational efficiency are two advantages of this utilization.
6. Data Analysis and Insights
Compared to previous approaches, LLMs can handle and interpret larger volumes of data more quickly and accurately. This greatly improves insights and data analysis. Products that many of us use on a daily basis, like the Google search engine, may even employ LLM.
Google uses LLMs to examine user behavior, identify patterns, and evaluate search results. Google enhances user experience across its search engine and other services and optimizes its AI algorithms with the use of such data analysis and insights.
Google can even forecast customer behavior and industry trends with the use of LLM, which enables them to provide more specialized advertising services. With the aid of accurate insights and analytics that facilitate data-driven decision-making, LLM may also be contributing to Google’s success in maintaining its competitiveness throughout the years.
7. Enhanced Customization in Customer Support
Better customer service customization enables companies to know how LLMs benefit businesses, assess consumer preferences, provide individualized interactions, and provide people with individualized information, services, or goods. Customers and the business both gain from this, as it speeds up consumer inquiries, increases customer satisfaction, and fosters loyalty.
Netflix is among the businesses that use this advantage with the aid of LLMs that examine user preferences and watching habits. Netflix uses this data to provide its 260 million subscribers with tailored content suggestions. With this degree of customization, Netflix subscribers may quickly locate entertainment that fits their tastes.
Users spend less time looking through information and more time watching as a result. Netflix maintains consumer satisfaction and entertainment in this manner. That’s a terrific method to maintain a large volume of returning users, which is crucial for new members. One advantage of Netflix’s LLM is that it consistently boosts income.
8. Human Resource Management
Automating different human resource procedures, large language models, and artificial intelligence may also improve human resource management. Everything from hiring to employment is covered by automated management. Additionally, it may assist employers in finding prospects and vice versa in the job-matching process.
An LLM on LinkedIn, for instance, evaluates millions of profiles and job postings to assist in suggesting the most suitable candidates. To pair the best matches on the site, it also computes key parameters like experience, abilities, and others.
Consequently, the hiring process is expedited, and the quality of the matches is improved. This shortens the time needed to pay experts to find matches and evaluate data. Moreover, LinkedIn’s job satisfaction and retention rates are increased by human resource management practices like this one. Artificial intelligence is transforming human resources by enabling LLMs to better analyze workforce trends and enhance employee experiences.
9. Competitive Advantage
From corporate data, even unstructured data, LLMs may extract useful insights. They do this quickly and precisely, enabling businesses to remain ahead of the competition, predict market trends, and make data-driven choices more quickly than ever before.
Businesses may employ LLMs to enhance the consumer experience in addition to enhancing goods and services. This raises consumer happiness, encourages repeat business, and even boosts income. One business that uses LLMs to simplify operations is AcmeTech, which offers it a major competitive edge.
This business uses LLMs to assess consumer sentiment, trends, and feedback on a big scale more quickly and with fewer errors. AcmeTech uses this data to customize its product recommendations and marketing tactics, improving customer happiness and marketing accuracy.
Difference Between Large Language Models and Generative AI
You may come across the statement that “not all generative AIs are LLMs, but every LLM is a generative AI.” It might sound a bit complicated at first, but let’s break it down to understand it more clearly. At the core of this statement is the idea that LLMs (large language models) are a specific type of generative AI, but not all generative AIs fall into the category of LLMs.
LLMs are designed primarily for understanding and generating human language. They excel in tasks such as language comprehension, answering questions, translation, summarization, and text generation, making them essential tools in natural language processing.
Recently, however, the benefits of large language models have expanded. They are no longer limited to processing and generating text alone. Many of the latest LLMs are multimodal, meaning they can process and generate not just text, but also images, videos, and even sound.
This expansion of their abilities demonstrates that LLMs are increasingly becoming versatile in their outputs, making them an integral part of generative AI models that span multiple media types. Thus, LLMs are a subset of the larger generative AI umbrella, which encompasses any model designed to create content, whether it’s text, images, music, or something else entirely.
On the other hand, the term “generative AI” is a broader concept that refers to various models and systems designed to generate new data or content. This includes techniques such as Generative Adversarial Networks (GANs) and Recurrent Neural Networks (RNNs). GANs, for instance, are typically used to generate images, while RNNs are better suited for generating music and sequential data.
This variety of models shows that generative AI doesn’t always rely on LLMs to produce creative outputs, but LLMs, due to their specialized capabilities in language, are indeed a crucial part of the generative AI landscape.
FAQ
Q: What is the training process for LLMs?
A: Using a technique known as self-supervised learning, large language models (LLMs) are trained on enormous text and code datasets. The model learns to predict the subsequent word or token in a sequence, and supervised learning is then used to fine-tune the model for particular applications.
Q: Beyond language, what do large language models learn?
A: Natural language processing has quickly made large language models (LLMs) a standard. It is well known that these models learn a wealth of linguistic information via extensive text training.
Q: Is a big language model capable of reasoning?
A: Despite not being specifically taught to reason, large language models (LLMs) have shown “emergent” behaviors that sometimes mimic reasoning.